Solving AI Energy Problems with Neuromorphic Technology
With the expansion of AI usage, the energy consumed by computers has been increasing explosively. TDK is developing a spin memristor, an analog memory element that electrically mimics the synapses in the human brain. Devices that utilize this technology, known as neuromorphic devices, are expected to be over 100 times more energy-efficient than conventional devices. This device technology can be manufactured using techniques similar to those currently used for MRAM*1 production. Leveraging its expertise in magnetic technology, developed through HDD heads and magnetic sensors, TDK aims to reduce the energy needed for AI and to discover new AI devices that can learn in real-time and adapt to their environment and users.
*1 MRAM:
Magnetoresistive Random Access Memory. Unlike conventional memory, data does not disappear (non-volatile). This allows for reduction in calculation and standby power as it can be quickly started even if the circuit power is turned off.
Contents
The rise of AI use and the energy problem
Today, AI-powered services are rapidly expanding in our daily lives, and news about AI is increasingly common. While current AI is primarily cloud-based, in the future, it is expected to grow into a vast market closer to the edge, more akin to human interaction. However, the development of the latest AI technologies requires substantial computational resources, leading to an explosive increase in global energy consumption, posing a new social challenge.
Therefore, to achieve widespread societal implementation of AI, a significant reduction in power consumption is essential. Until now, the growth of technology has been supported by the miniaturization of semiconductors and the evolution of digital architectures. However, as we approach the limits of Moore's Law*2 and the realization of the Von Neumann bottleneck*3, this approach is reaching its limits, and a strong demand for new solutions is emerging.
*2 Moore's law:
The rule of thumb that the integration density of semiconductor circuits doubles every 18 (or 24) months. The evolution of miniaturization technology has supported the advancement of today's semiconductor industry.
*3 Von Neumann bottleneck:
One of the causes that limit the processing power of computers. It refers to the limit of processing power that arises from the restriction on data transfer between the CPU and memory.
Neuromorphic devices mimicking human brain functions
Neuromorphic devices, which mimic the functionality of the human brain, are emerging as innovative technologies to address the power consumption issues of AI. These devices draw inspiration from the brain's structure, operating on approximately 20W—just a fraction of the power required by current digital AI computations—yet capable of making complex decisions. The human brain's intricate network of synapses and neurons is electrically replicated in neuromorphic devices, with memristors playing a pivotal role. These components, whose conductivity and resistance vary with the charge passed through them, emulate the function of synapses. By connecting multiple memristors to form an array network, neuromorphic devices can approximate the brain's signal processing, significantly reducing power consumption.
Challenges with conventional memristor devices
Historically, memristor elements such as ReRAM*4 and PCM*5 have been explored for their potential in neuromorphic devices. However, their complex response behaviors and the tendency for resistance values to drift over time have presented challenges. These characteristics make them unsuitable for analog use in neuromorphic devices, thus requiring circuit and algorithmic corrections to compensate. This has led to complexities in circuit design and limitations in real-time learning, highlighting the need for the development of more synapse-compatible elements.
*4 ReRAM: Resistive random access memory
*5 PCM:Phase Change Memory
Features and advantages of TDK spin memristors
TDK's spin memristor distinguishes itself by leveraging magnetic resistance effects, a principle derived from the company's experience with HDD heads and magnetic sensors. This technology combines the benefits of data retention and controllability, facilitating the realization of low-power neuromorphic devices with simpler circuits. Furthermore, the superior controllability allows for on-chip AI learning without relying on the cloud, a task previously challenging with other elements. TDK is advancing the development of neuromorphic device chips using spin memristors, aiming for technology demonstration at the chip level.
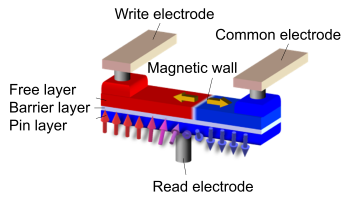
A three-terminal magnetic resistance effect element. It consists of a free layer, a barrier layer, and a pinned layer The resistance value changes depending on the position of the magnetic wall. Writing is performed by horizontal current, and reading is performed by vertical current."
The future of ultra-low power AI devices
The deployment of AI for advanced information processing indispensably requires substantial computational resources and energy. TDK's spin memristor represents a significant stride towards reducing AI's power consumption, addressing the societal challenge of increased energy demands accompanying AI advancements. Utilizing the stable characteristics of spin memristors, it's possible to enable both learning and inference functionalities on-chip. This shifts the paradigm from humans adapting to pre-trained AI models to AI that adapts and evolves according to its environment. TDK plans to integrate this technology with its sensor expertise, paving the way for smart sensors that continually offer optimal information tailored to individuals and their surroundings, enhancing life quality through closer AI interaction.
Regarding inquiries, materials, etc.
-
Ultrasensitive magnetic sensor detecting one ten-millionth of geomagnetism
Shieldless and easy-to-use device capable of detecting extremely weak magnetic field. -
Thin-film Capacitors Designed for Integration in Circuit Boards, Thickness of 50μm or Less, Flexible and Crack Resistant
Innovations for decoupling capacitors embedded within the circuit board substrate below the LSI chip. -
New Ag-Stacked Transparent Conductive Film
A functional film that contributes to the conservation and creation of energy. -
TDK Biomagnetic Sensor: Effective Alternative to SQUID (Superconducting Quantum Interference Device)
Capable of detecting extremely weak biomagnetic signals at room temperature. The world's first ambient temperature sensor to visualize the heart's magnetic field distribution. -
Optical Components Supporting Next Generation Optical Communication / Optical Transmission System
Backgrounds and technologies that support TDK's development of optical communication components and modules